Women in machine learning and the neural information processing systems conference 2015
December 7th saw the start of the 10th annual Women in Machine Learning workshop (WiML 2015) in Montreal, Canada, which coincides with the 29th Annual conference on Neural Information Processing Systems conference (NIPS 2015). I was awarded a travel scholarship to attend this event and to present my BSc. final year research topic “Content Extraction and Context Inference based Information Retrieval” as a poster presentation.
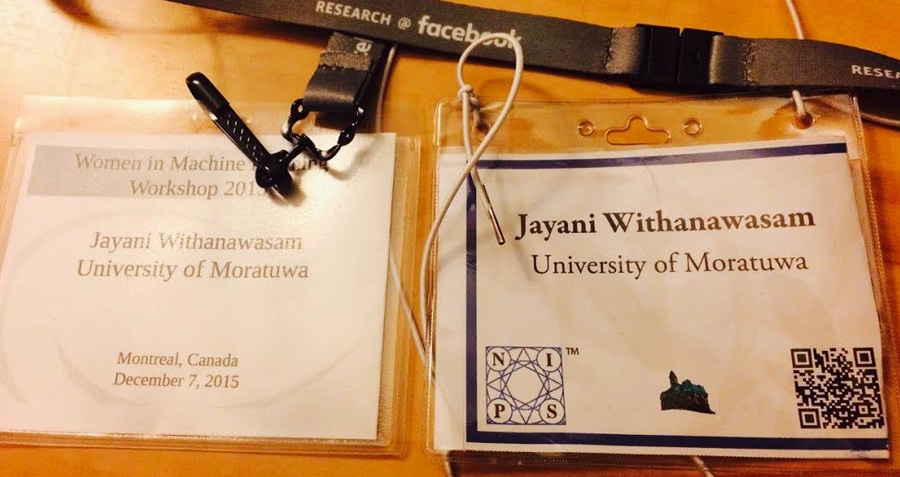
Women in Machine Learning (WiML) is a technical workshop, which gives an opportunity for female machine learning researchers, such as research scientists and graduate students to meet, share ideas and learn interactively. The two day long WiML 2015 workshop included an NVIDIA deep learning tutorial, a Facebook Lean in circle event and a combination of invited talks as well as contributed talks by Female machine learning researchers.
During the NVIDIA deep learning tutorial, the importance of using GPUs to speed up neural network computations was discussed. It was followed by a demonstration of NVIDIA DIGITS, the interactive deep learning GPU system. The application of deep learning for image classification was also discussed.
Lean In is a non-profit organisation to empower women to achieve their ambitions by sharing each other’s life experiences. We had a Lean In event, sponsored by Facebook prior to WiML workshop and it was a great opportunity to share our experiences with fellow WiML attendees.
The Invited and contributed talks comprised of various research findings and applications of machine learning techniques such as reinforcement learning, classification and recommendations. Another highlight in WiML workshop was the career advice session tables, which focused on the diverse challenges women face while engaging in research such as long term planning, work life balance, keeping up with academia while in the industry and self-advocacy.
My Poster Presentation
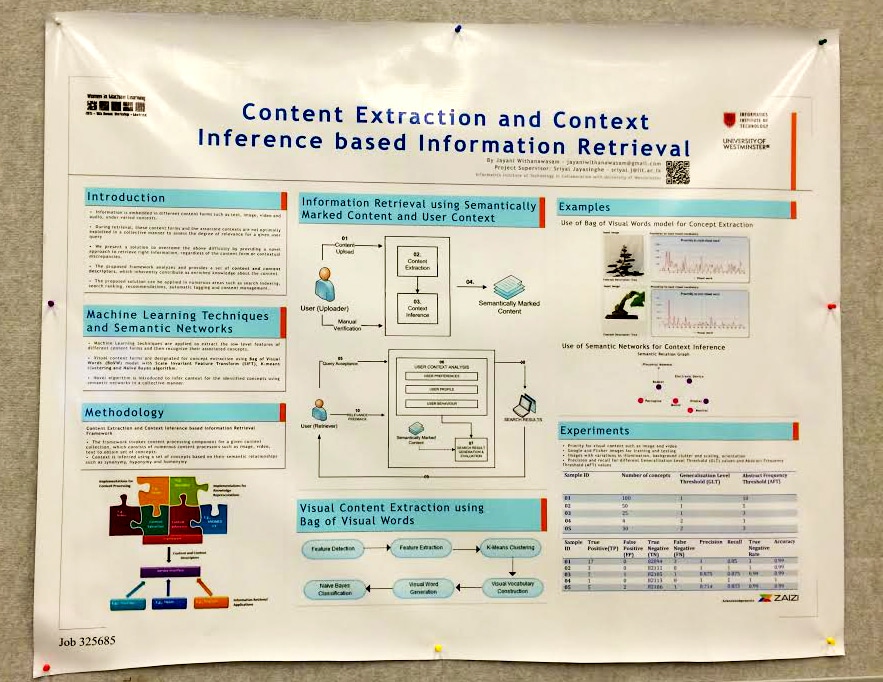
During the poster sessions, I presented my idea that proposed a framework-based approach using machine learning and knowledge representation where correct information can be retrieved regardless of the content format or contextual discrepancies. Given that information can be embedded as any content format such as images, video, audio and text, the proposed framework analyses and provides a set of content and context descriptors, which can be used in any information retrieval application.
Neural Information Processing Systems Conference
After presenting in WiML 2015, I was fortunate enough to attend NIPS 2015, which one of the most prestigious conferences on machine learning in the world. NIPS is a machine learning and computational neuroscience conference that contains invited talks and oral and poster presentations of cutting edge research done by top-notch universities and industries.
In NIPS 2015, novel machine learning algorithms, enhancements or optimizations (E.g., memory consumption, computation time, scalability) in existing machine learning algorithms and theoretical analysis of machine learning algorithms were presented. Highlighted areas of research were low-level computer vision and image processing (image segmentation, scene labeling, object detection) and natural language processing (grammar understanding, sequence modeling). Further, there were papers presented on decision-making and medical diagnosis as well. It was strongly evident that deep learning algorithms such as Convolutional Neural Networks (CNNs) have the edge over other algorithms in majority of applications.
Last but not least, since the event was held in Montreal (a predominantly French city in Quebec, Canada), whenever I found time I used it to wander around Old Montreal streets , that gave a European sensation amidst Northern American cultures which was a truly unique experience.
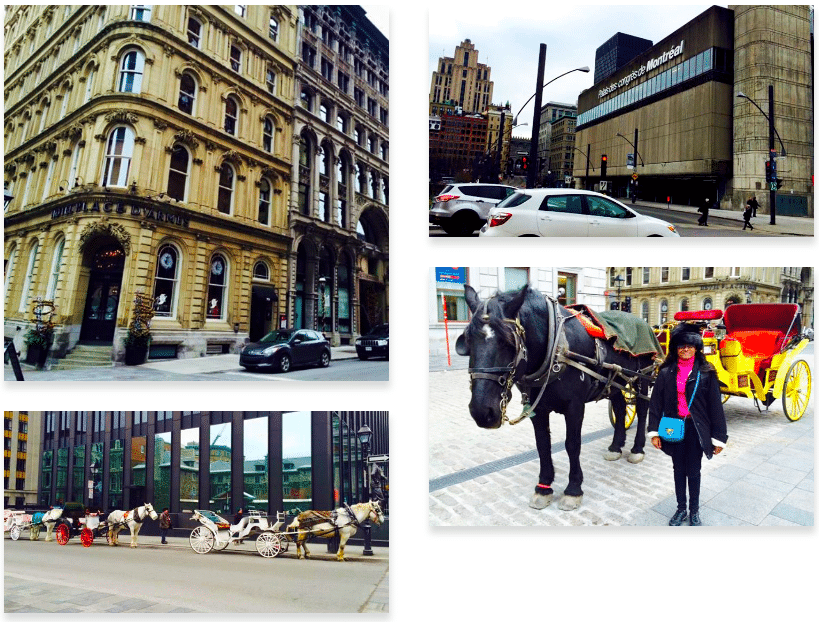
Latest content
-
New report shows how the Labour government can avoid the policy-delivery gap
Published on: 17 July, 2024 -
Digital-first governance: Reflections on the first week of a Labour government
Published on: 12 July, 2024 -
Why digital deliverability must be a key test for policy post-election
Published on: 10 July, 2024 -
Bringing an SME perspective to government’s Code of Practice for Software Vendors
Published on: 4 July, 2024